Learning to Design Analog Circuits to Meet Threshold Specifications
Dmitrii Krylov, Pooya Khajeh, Junhan Ouyang, Thomas Reeves, Tongkai Liu, Hiba Ajmal, Hamidreza Aghasi, and Roy Fox
40th International Conference on Machine Learning (ICML), 2023
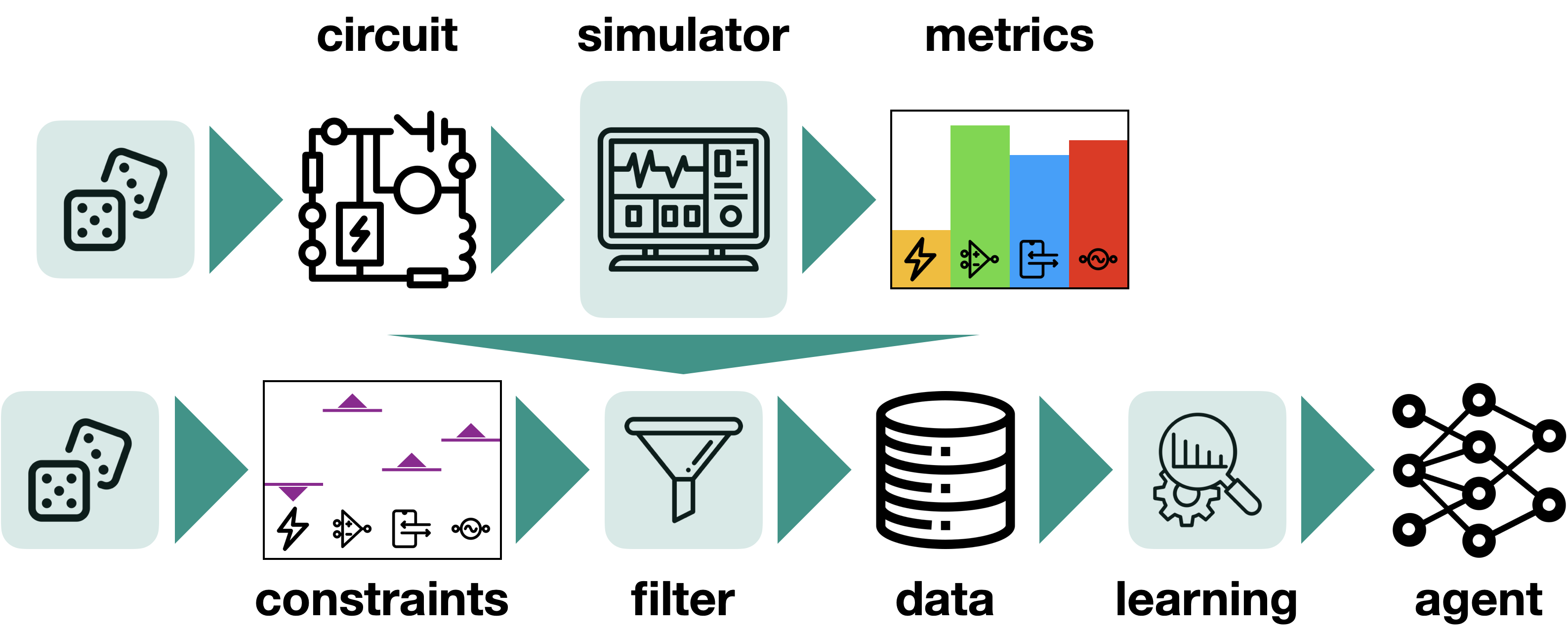
Automated design of analog and radio-frequency circuits using supervised or reinforcement learning from simulation data has recently been studied as an alternative to manual expert design. It is straightforward for a design agent to learn an inverse function from desired performance metrics to circuit parameters. However, it is more common for a user to have threshold performance criteria rather than an exact target vector of feasible performance measures. In this work, we propose a method for generating from simulation data a dataset on which a system can be trained via supervised learning to design circuits to meet threshold specifications. We moreover perform the to-date most extensive evaluation of automated analog circuit design, including experimenting in a significantly more diverse set of circuits than in prior work, covering linear, nonlinear, and autonomous circuit configurations, and show that our method consistently reaches success rate better than 90% at 5% error margin, while also improving data efficiency by upward of an order of magnitude. A demo of this system is available at