Make the Pertinent Salient: Task-Relevant Reconstruction for Visual Control with Distraction
Kyungmin Kim, Charless Fowlkes, and Roy Fox
Training Agents with Foundation Models workshop (TAFM @ RLC), 2024
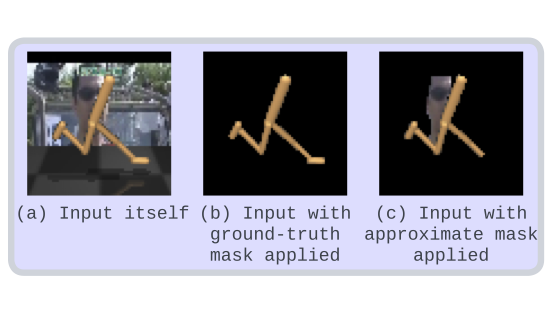
Model-Based Reinforcement Learning (MBRL) has been a powerful tool for visual control tasks. Despite improved data efficiency, it remains challenging to use MBRL to train agents with generalizable perception. Training with visual distractions is particularly difficult due to the high variation they introduce to representation learning. Building on Dreamer, a popular MBRL method, we propose a simple yet effective auxiliary task — to reconstruct task-relevant components only. Our method, Segmentation Dreamer (SD), works either with ground-truth masks or by leveraging potentially error-prone segmentation foundation models. In DeepMind Control suite tasks with distraction, SD achieves significantly better sample efficiency and greater final performance than comparable methods. SD is especially helpful in a sparse reward task otherwise unsolvable by prior work, enabling the training of a visually robust agent without the need for extensive reward engineering.